As healthcare providers strive for enhanced service delivery and greater efficiency, embracing digital transformation is increasingly crucial. A pivotal element of this digital shift involves recognizing the significance of data and utilizing big data analytics to harness its full potential.
Among the various categories of data analytics in healthcare, predictive analytics stands out for its capacity to create substantial value. Unlike traditional analytics that merely describe past events and their causes, predictive analytics offers a forward-looking perspective by analyzing trends in historical data to predict future outcomes.
This predictive capability is transformative for healthcare organizations transitioning from a reactive to a proactive approach. However, before implementing this advanced analytical tool, it is essential to identify specific, relevant use cases.
In this detailed guide, Informessor will present 10 key use cases for predictive analytics that health systems can incorporate into their strategies to not only anticipate future trends but also to strategically prepare for them. This exploration will delve into the strategic advantages of predictive analytics and how it can fundamentally redefine the landscape of healthcare operations and patient care.
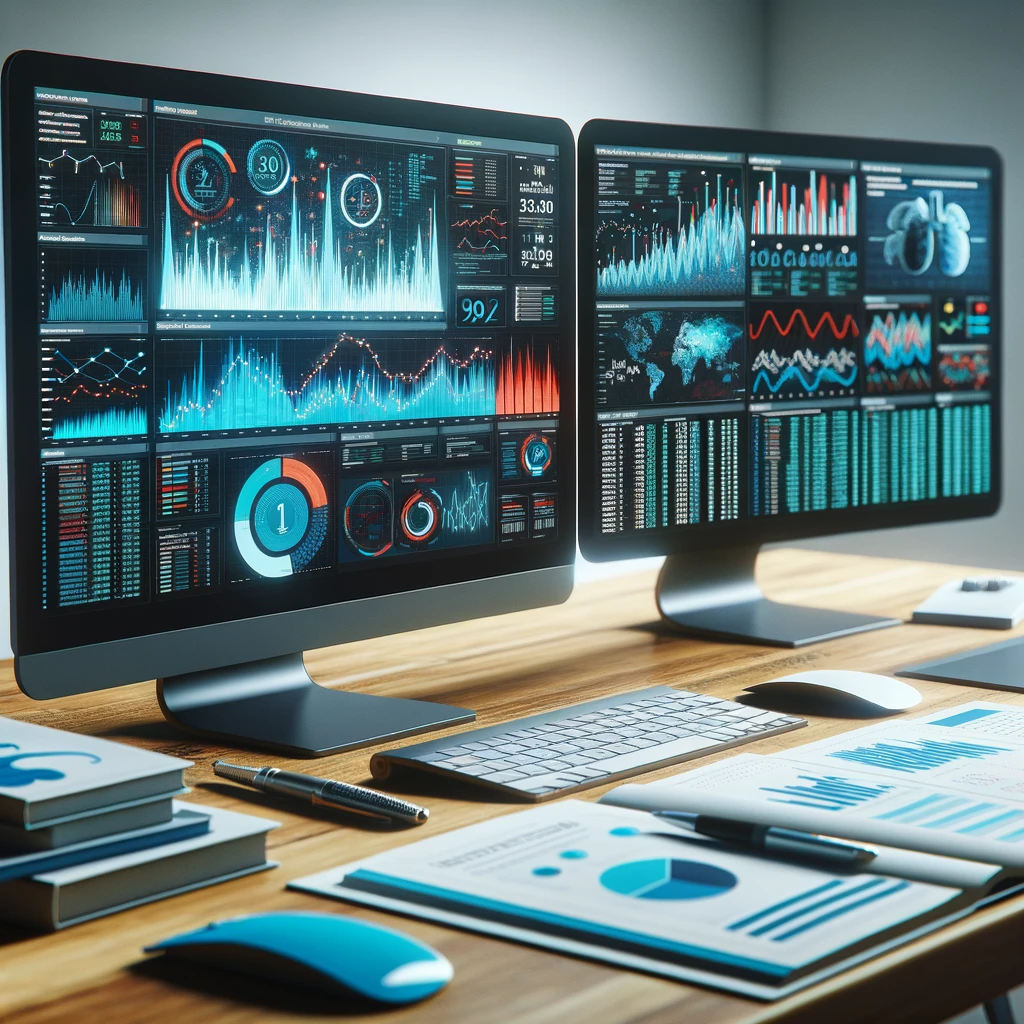
1. Early Disease Detection
Predictive analytics is revolutionizing the field of healthcare through its profound impact on early disease detection. By harnessing vast amounts of medical data—from genetic information and family history to lifestyle choices and previous health records—this technology can identify patterns that precede the onset of conditions such as diabetes, heart disease, or even cancer. What makes predictive analytics particularly exciting is its ability to sift through data that might appear inconsequential at a glance but can reveal critical insights when analyzed in aggregate.
The process involves sophisticated algorithms and machine learning models that learn from existing data, improving their accuracy over time as they are fed more information. For instance, by analyzing retinal images, predictive models can detect early signs of diabetic retinopathy, enabling earlier intervention before the condition progresses. Similarly, algorithms can evaluate patterns in skin images to flag early stages of melanoma, potentially saving lives through earlier treatment.
The impact of early disease detection is monumental. It not only enhances the efficacy of treatments but also significantly reduces healthcare costs by curbing the need for extensive medical interventions required at more advanced stages. Moreover, patients benefit from a higher quality of life with minimal disease complications, underscoring the transformative power of predictive analytics in preemptive health management. This shift towards anticipatory healthcare is not just a technological achievement; it’s a new paradigm that places as much emphasis on preventing illness as it does on curing it.
2. Patient Risk Satisfaction
Patient risk stratification, facilitated by predictive analytics, is another transformative application in healthcare that is optimizing how care is delivered. This approach involves analyzing layers of patient data—including demographic information, clinical histories, and even real-time health metrics—to categorize patients based on their risk of developing certain conditions or experiencing adverse health events. This stratification enables healthcare providers to tailor care plans that are both effective and efficient, targeting resources to those who need them most.
For example, a patient with a high risk of cardiovascular disease might be identified based on a combination of high blood pressure readings, cholesterol levels, and a family history of the disease. Healthcare providers can then prioritize interventions for this patient, such as prescribing medication, recommending lifestyle changes, and scheduling regular monitoring. This proactive approach not only improves patient outcomes but also enhances the overall management of healthcare resources by preventing hospital re-admissions and reducing the incidence of emergency interventions.
The beauty of patient risk stratification lies in its precision. Rather than applying a one-size-fits-all approach, it allows for personalization of care that respects individual health trajectories and needs. The method is dynamic, with risk profiles updated continuously as new data becomes available, ensuring that the care plans remain relevant and responsive. This targeted approach not only fosters better patient engagement and satisfaction but also propels a shift towards a more sustainable healthcare system, where resource allocation is driven by data-informed decisions, ultimately leading to more resilient health outcomes.
3. Personalized Treatment Plans
Predictive analytics plays a crucial role in creating personalized treatment plans that cater to the unique genetic and environmental factors of each patient. This tailored approach is far from the traditional one-size-fits-all strategy; it uses complex algorithms to analyze a vast array of patient-specific data, including genetic profiles, past medical history, and lifestyle factors. The outcome is a treatment plan that not only targets the ailment more precisely but also minimizes potential side effects.
For example, in cancer treatment, predictive analytics can be used to determine which chemotherapy drugs a patient is most likely to respond to, based on the genetic mutations present in their tumors. This not only increases the effectiveness of the treatment but often reduces the patient’s exposure to potentially harmful side effects. Similarly, in chronic diseases like diabetes, predictive models help forecast how different individuals might react to various medications or diet changes, enabling more accurate and effective management of the disease.
The benefits of personalized treatment plans extend beyond improved health outcomes. They also enhance patient satisfaction by involving patients in their treatment processes, thereby fostering a sense of control and partnership in managing their health. This personalized care approach is not just transforming patient experiences; it is setting new standards in healthcare, making it more responsive, effective, and deeply attuned to the needs of individual patients.
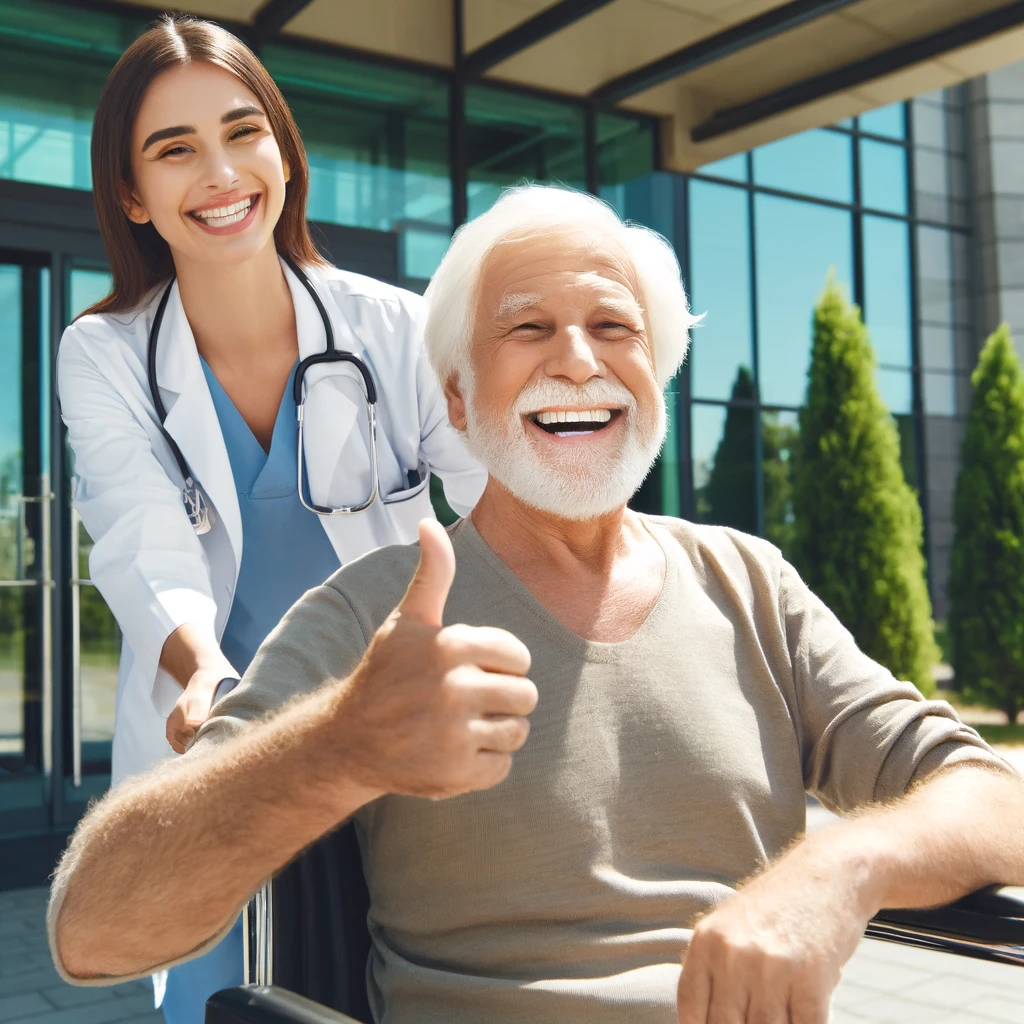
4. Reduction in Readmissions
Reducing hospital readmissions is a critical objective for healthcare systems, not only because of the high costs associated but also for improving the quality of care. Predictive analytics serves as a powerful tool in this arena by identifying patients who are at high risk of readmission before they even leave the hospital. By leveraging data such as previous hospital stays, medication records, lab results, and even socio-economic factors, healthcare providers can predict which patients might face difficulties once discharged.
This predictive insight allows for the implementation of customized interventions aimed at preventing readmissions. For instance, a patient recovering from heart surgery might be identified as high-risk due to factors like age, complexity of surgery, and lack of family support at home. In response, the hospital can arrange for enhanced follow-up care, at-home nursing support, or even remote monitoring to ensure compliance with post-operative instructions. These steps help address potential complications early, reducing the likelihood of the patient returning to the hospital.
The impact of using predictive analytics to reduce readmissions is profound. It not only saves significant healthcare costs but also signals a shift towards a more preventative care model, where resources are allocated more efficiently, and patient outcomes are markedly improved. In this system, hospitals are not just healing centers but also partners in long-term health management, actively working to ensure ongoing wellness for their patients.
5. Chronic Disease Management
Effective management of chronic diseases is a cornerstone of sustainable healthcare, and predictive analytics is reshaping how these conditions are handled. By continuously monitoring health data and predicting future health trends, predictive analytics allows healthcare providers to preemptively adjust treatment plans, manage symptoms more effectively, and prevent disease progression. This proactive approach not only enhances patient quality of life but also reduces the burden on healthcare systems.
For instance, consider the management of Type 2 diabetes—a condition that affects millions worldwide. Predictive analytics can analyze patterns from glucose readings, diet logs, physical activity, and even patient-reported symptoms to forecast potential spikes or drops in blood sugar levels. This enables timely intervention, such as adjusting insulin doses or dietary recommendations before the patient experiences a critical event. The ongoing monitoring and predictive insights help maintain optimal glucose levels and minimize complications, such as neuropathy or retinopathy, that can arise from poorly managed diabetes.
The integration of predictive analytics into chronic disease management is transforming patient care from reactive to proactive, significantly impacting long-term health outcomes. Patients are equipped with personalized, data-driven insights that empower them to take control of their health, while healthcare providers can offer more precise and effective treatments based on predicted disease trajectories. This smarter approach to healthcare not only optimizes resource use but also aligns closely with the goals of patient-centered care, ultimately fostering a healthier population.
6. Medication Adherence
Medication adherence is a critical factor in the effectiveness of treatment regimens, particularly for chronic conditions where long-term medication use is common. Predictive analytics is becoming an indispensable tool in addressing the challenge of non-adherence, which can lead to poorer health outcomes and increased healthcare costs. By identifying patients who are at risk of not following their prescribed medication plans, interventions can be timely and specifically tailored to individual needs.
Predictive models use various data points such as patient demographics, past medication adherence history, social determinants of health, and even psychological factors to assess the risk of non-adherence. For example, a patient with a complex medication regimen, limited social support, and history of depression may be identified as at high risk for non-adherence. Healthcare providers can then implement targeted strategies, such as simplified medication schedules, regular follow-up calls, or digital reminders, to enhance adherence.
Moreover, predictive analytics can help tailor communication and education efforts to match the patient’s specific circumstances and preferences, increasing their engagement and commitment to the treatment plan. This personalized intervention does more than just improve adherence; it builds a supportive relationship between patients and healthcare providers, enhancing trust and encouraging open communication about treatment challenges.
By leveraging predictive analytics to tackle medication adherence, healthcare systems not only improve individual patient outcomes but also contribute to more efficient and effective healthcare delivery. These analytics-driven interventions are a testament to how data can be used to not only understand but also actively improve patient health behaviors and treatment success.
7. Fraud Detection
In any field and especially in healthcare, financial integrity is paramount, and predictive analytics has emerged as a vital tool for detecting and preventing fraud. Healthcare fraud, which can range from false billing to illegal kickbacks, significantly drains resources that could otherwise be allocated to patient care. Predictive analytics combats this issue by scrutinizing patterns in billing and claims data to identify anomalies that may suggest fraudulent activity.
This approach involves algorithms that are trained on historical billing data, including legitimate and fraudulent claims. By learning what typical fraudulent activities look like—such as billing for services not rendered or exaggerating the level of services provided—these models can flag similar occurrences in new data. For example, a sudden increase in billing for a particular procedure that doesn’t align with the patient’s medical history or geographic trends can trigger an alert for closer examination.
The use of predictive analytics for fraud detection not only helps recover costs but also deters potential fraudsters by increasing the likelihood of detection. This proactive stance helps maintain the financial health of healthcare institutions and ensures that funds are used for genuine healthcare services. Moreover, reducing fraud contributes to lower healthcare costs overall, benefiting patients, providers, and insurers alike. As predictive analytics technology advances, its role in safeguarding the financial aspects of healthcare continues to grow, making it an indispensable asset in the ongoing fight against fraud.
8. Surgical Outcomes Prediction
Predictive analytics is transforming surgical care by providing insights that enhance patient safety and improve surgical outcomes. By analyzing a wealth of preoperative data, including patient health records, previous surgical outcomes, and even genetic information, predictive models can forecast the likely results of surgeries. This foresight enables healthcare providers to make better-informed decisions about whether to proceed with surgery, what precautions to take, and how to tailor post-operative care to individual patients.
The use of predictive analytics in surgery goes beyond mere risk assessment. It includes detailed predictions about a range of potential outcomes, such as the likelihood of complications, the duration of hospital stay, and the speed of recovery. For example, in orthopedic surgery, predictive models might analyze factors such as age, body mass index, and pre-existing conditions to predict the patient’s rehabilitation process and potential challenges.
This predictive capability not only enhances the precision of surgical planning but also improves patient consultations. Surgeons can discuss potential risks and outcomes with patients more effectively, leading to informed consent based on comprehensive data-driven insights. Additionally, by anticipating potential complications, healthcare teams can strategize preemptive interventions, thus reducing the incidence of adverse events and enhancing overall patient care.
Ultimately, the integration of predictive analytics into surgical practices exemplifies the shift towards more personalized and preemptive healthcare. By accurately predicting surgical outcomes, healthcare providers can optimize both the effectiveness of surgeries and the allocation of medical resources, thereby elevating the standard of care provided to patients.
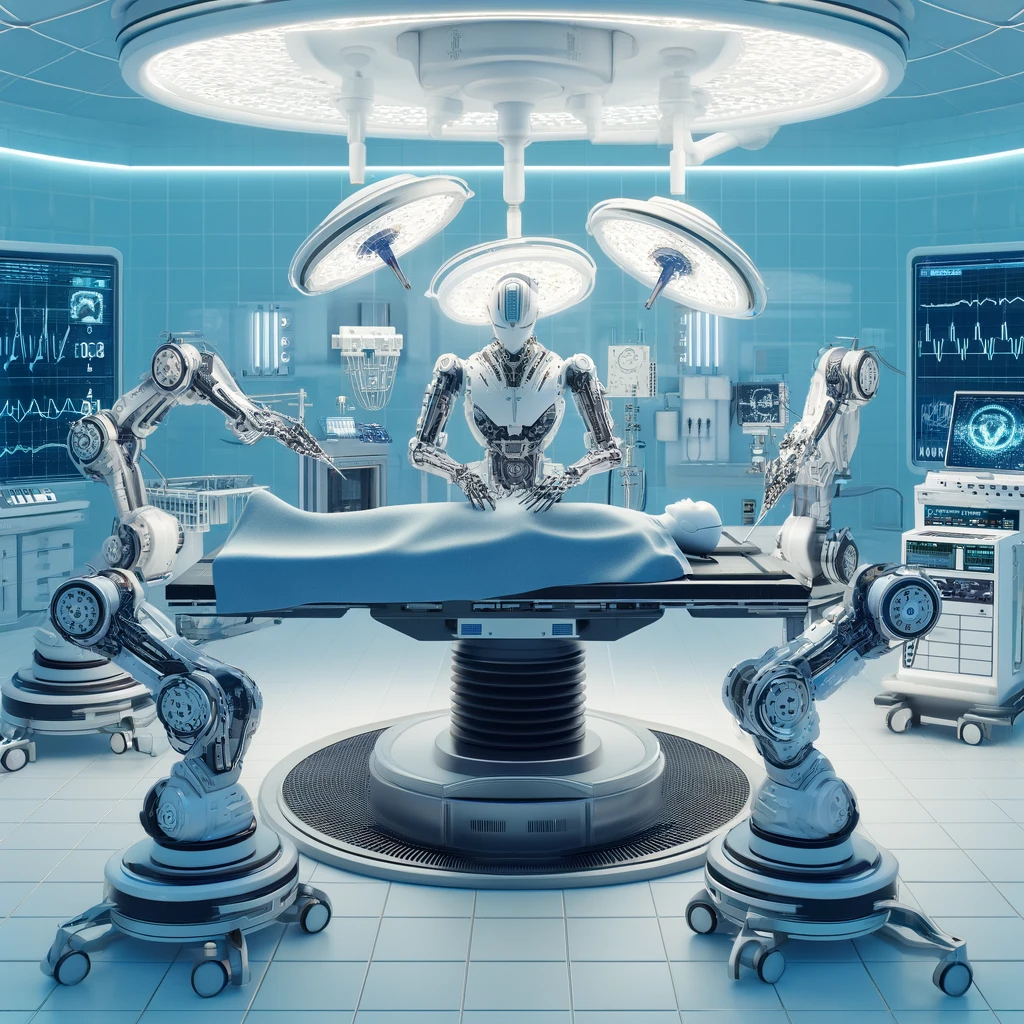
9. Patient Flow Optimization
Optimizing patient flow within hospitals is critical to reducing wait times and enhancing the quality of care. Predictive analytics plays a pivotal role in achieving these goals by forecasting the number of patient admissions, discharges, and transfers, thereby enabling more effective resource allocation and scheduling. This strategic foresight helps hospitals manage their staffing needs, prepare for peak periods, and ensure that patients receive timely care.
For instance, predictive models can analyze historical admission rates, seasonal trends, local events, and even weather patterns to predict patient influx. With this information, hospital administrators can adjust staffing levels, optimize bed availability, and streamline operations to handle the anticipated patient load. This is particularly crucial in departments like emergency rooms, where predicting high-traffic periods can significantly impact the efficiency of care delivery.
Additionally, improved patient flow leads to reduced congestion in critical areas of the hospital, minimizing the risk of infections and increasing patient satisfaction. It also facilitates better communication between departments, ensuring that transitions from surgery to recovery rooms, for instance, are smooth and timely. By leveraging predictive analytics to manage patient flow, hospitals not only enhance operational efficiency but also elevate the overall patient experience, making healthcare more responsive and patient-centric.
10. Cost Management
Predictive analytics offers a powerful tool for financial stewardship within the healthcare sector. By forecasting future spending patterns, patient care needs, and resource requirements, predictive analytics helps healthcare organizations plan and allocate budgets more effectively, ultimately controlling costs without compromising the quality of care.
Predictive models can analyze various data points, such as historical treatment costs, patient outcomes, and resource utilization rates, to identify areas where spending can be optimized. For example, by predicting which patients are at higher risk for readmission, hospitals can implement targeted preventive measures that reduce costly readmissions and enhance patient health outcomes. Similarly, predictive analytics can help pharmaceutical inventory management by forecasting medication needs, thereby minimizing waste due to overstocking or expirations.
The strategic use of predictive analytics extends to negotiating better rates with suppliers, optimizing insurance claim management, and even setting more accurate pricing models for services. This proactive approach to cost management not only ensures financial health for healthcare providers but also contributes to the affordability of healthcare for patients. As healthcare organizations become more adept at using these predictive insights, they can achieve a balance between cost containment and high-quality patient care, proving that financial prudence and excellent healthcare can go hand in hand.
Related Articles:
The Important Role of Data Analytics in Healthcare
The Role of SQL in Healthcare: Enhancing Data Analysis for Improved Patient Outcomes
Predictive Analytics in Healthcare: Anticipating Needs and Improving Outcomes