The Intersection of AI and Pharmaceuticals
Machine learning is revolutionizing the pharmaceutical industry, redefining how drugs are discovered, developed, and brought to market. Traditional drug discovery methods are notoriously time-intensive and costly. On average, it takes around 10-15 years and approximately $2.6 billion to develop a new drug, according to the Pharmaceutical Research and Manufacturers of America, PhRMA. These methods often rely on trial and error processes and manual screening, which can be inefficient and prone to failure. This is where machine learning comes in, an innovative technology poised to transform this issue.
Machine learning excels at analyzing large datasets to uncover patterns and insights that humans might overlook. In drug discovery, ML algorithms can process vast libraries of molecular data, predict potential drug candidates, and even simulate how these molecules interact with biological targets. For instance, companies like DeepMind have made headlines with AlphaFold, a tool capable of predicting protein structures with great accuracy. This is an achievement that significantly accelerates the early phases of drug discovery.
Moreover, ML isn’t limited to just discovery as it also enhances development. By analyzing clinical trial data in real time, ML models can identify patient subgroups most likely to benefit from a drug, optimize trial designs, and predict potential adverse effects. This reduces the risk of costly trial failures and accelerates regulatory approvals.
As the pharmaceutical industry embraces AI and ML, these tools are not just a luxury but a necessity in navigating the complexities of modern medicine. In this article, we’ll explore how machine learning is shaping the future of drug discovery and development, bringing hope for faster, safer, and more personalized medical solutions.
By leveraging these cutting-edge technologies, pharmaceutical companies can meet the growing demand for innovative treatments while reducing costs and timelines, making this a true game-changer in healthcare.
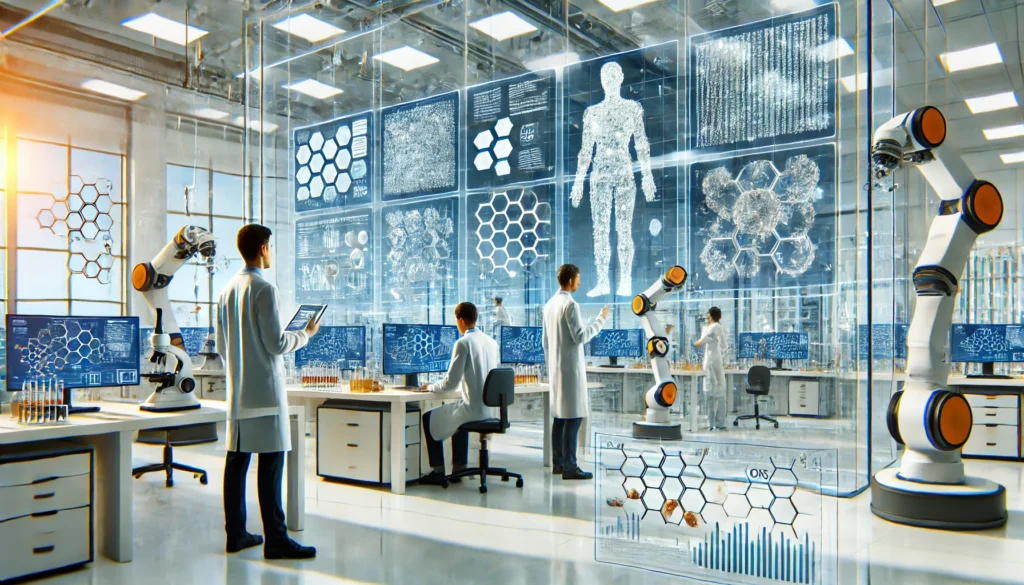
Accelerating Drug Discovery
The discovery of new drugs is one of the most challenging and expensive stages in pharmaceutical development. Machine learning is changing the game by enabling researchers to analyze vast datasets and identify promising drug candidates with unprecedented speed and accuracy.
One of the key ML methods in drug discovery is predictive modeling, where algorithms analyze patterns in biological and chemical data to forecast the efficacy and safety of potential drugs. For example, ML can predict how a molecule will interact with a specific protein target, dramatically reducing the need for costly lab experiments. This predictive capability enables researchers to focus resources on the most promising candidates.
Virtual screening is another ML-driven approach revolutionizing the field. Algorithms sift through extensive libraries of molecular structures, scoring and ranking compounds based on their likelihood to bind effectively to a biological target. Compared to traditional high-throughput screening methods, which require physical testing of thousands of compounds, virtual screening is faster and more cost-efficient.
ML also facilitates de novo drug design, where algorithms generate entirely new molecules optimized for specific biological functions. By leveraging generative models, such as deep learning, researchers can design novel compounds tailored to combat diseases more effectively. Companies like Insilico Medicine have used this approach to identify potential drugs for fibrosis and other conditions, compressing years of work into months.
These innovations have been reshaping the healthcare industry. With ML, the drug discovery process becomes more focused, reducing the average time from target identification to lead candidate selection by months, if not years.
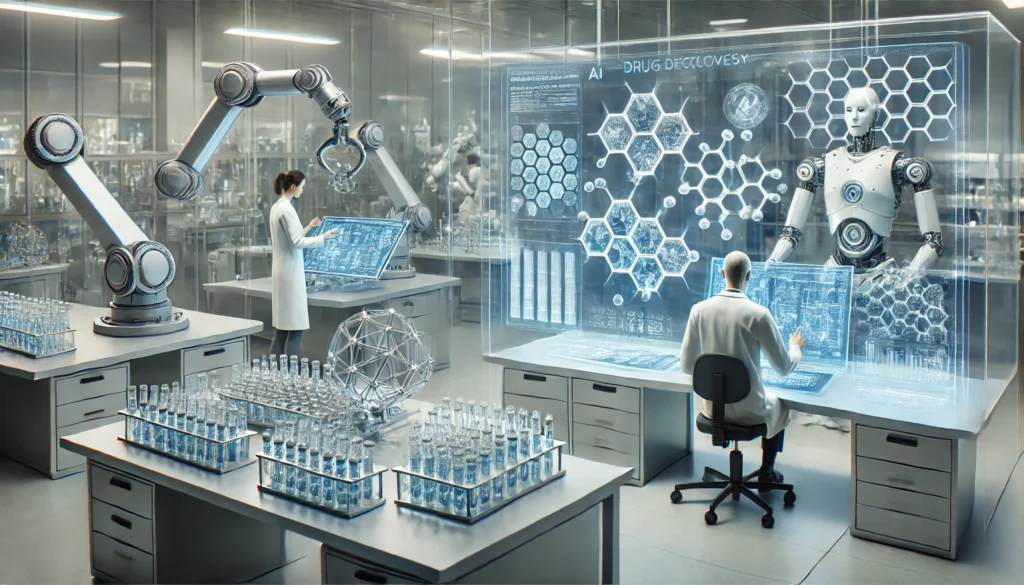
Optimizing Clinical Trials
Clinical trials are critical to bringing new drugs to market, but they are often plagued by inefficiencies. According to the Biotechnology Innovation Organization, only 12% of drugs entering clinical trials eventually receive FDA approval, with most failures attributed to issues like poor patient selection or safety concerns. Machine learning is transforming clinical trials by addressing these challenges, improving efficiency, reducing costs, and accelerating drug approvals.
One of the most impactful applications of ML in clinical trials is patient selection. Identifying the right participants for a trial is critical for accurate results. ML algorithms analyze electronic health records (EHRs), genetic profiles, and even social determinants of health to match patients with trials most suited to their specific conditions. This targeted approach not only increases the likelihood of trial success but also improves patient outcomes.
Real-time trial monitoring is another area where ML shines. Usually, monitoring trial progress requires manual data collection and analysis, which is time-consuming and prone to error. ML-powered tools can flag irregularities in real time, ensuring that adverse events are promptly addressed. For instance, IBM Watson Health’s ML solutions have been used to monitor trial data, identifying trends and outliers that would otherwise go unnoticed.
ML also enables predictive analysis to forecast outcomes and optimize trial designs. By simulating trial scenarios using historical and synthetic data, researchers can identify potential risks, predict drug efficacy, and refine trial parameters before they even begin. AstraZeneca has used ML to reduce trial delays and optimize resource allocation, demonstrating how AI can lead to quicker approvals.
By leveraging machine learning, pharmaceutical companies can minimize risks, improve trial efficiency, and bring life-saving therapies to the market faster.
Personalized Medicine and Drug Repurposing
In recent years, the one-size-fits-all approach to medicine has opened the path to personalized medicine, where treatments are tailored to the unique genetic, lifestyle, and medical profiles of individual patients. Machine learning plays a pivotal role in this transformation, enabling healthcare providers and researchers to deliver more effective and targeted therapies.
Through genomic analysis, ML algorithms can identify genetic variations that influence how a patient responds to specific drugs. For instance, ML models can analyze sequencing data to predict whether a patient carries genetic markers for drug resistance or heightened sensitivity. The insights allow clinicians to select therapies that maximize efficacy while minimizing adverse effects.
ML also excels in drug repurposing, the process of finding new uses for existing medications. By analyzing vast datasets of clinical trials, real-world evidence, and molecular interactions, ML can uncover hidden connections between drugs and diseases. A notable example is the repurposing of remdesivir during the COVID-19 pandemic, where data-driven approaches identified its potential as an antiviral therapy.
Furthermore, ML expedites this process by running simulations and predicting outcomes, bypassing the need for exhaustive lab experiments. This rapid identification of repurposing opportunities not only saves time and resources but also addresses unmet medical needs in areas like rare diseases and neglected conditions.
The fusion of ML with personalized medicine and drug repurposing marks a new frontier in healthcare. By aligning treatments with individual needs and uncovering novel therapeutic applications, machine learning is helping to redefine patient care and improve outcomes on a global scale.
Challenges and Future Prospects
Despite its transformative potential, machine learning in drug discovery and development faces significant challenges that hinder its full adoption. The biggest challenge among these is data quality and availability. The effectiveness of ML models relies heavily on the accuracy and diversity of the data they are trained on. However, healthcare data is often messy, inconsistent, and riddled with privacy constraints. Integrating datasets from various sources like electronic health records, clinical trials, and genomics requires sophisticated data harmonization techniques, which remain a bottleneck.
Regulatory hurdles further complicate the landscape. Drug development is a highly regulated field, and ML models must meet stringent standards to ensure safety and efficacy. Regulatory bodies like the FDA are still in the early stages of establishing clear frameworks for evaluating AI-driven solutions, creating uncertainty for pharmaceutical companies.
Ethical considerations are another critical barrier. Issues like data privacy, bias in algorithms, and the transparency of ML decisions (known as explainability) raise important questions about fairness and accountability. Without addressing these concerns, public trust and widespread adoption of ML in healthcare could be compromised.
However, the future looks promising. Advances in explainable AI (XAI) aim to make ML models more interpretable, ensuring stakeholders understand how decisions are made. Additionally, innovations in federated learning allow ML algorithms to train on decentralized datasets while preserving data privacy, mitigating some of the challenges tied to data sharing.
As the pharmaceutical industry continues to evolve, collaborations between tech companies, regulators, and healthcare providers are accelerating the integration of ML. With these advancements, machine learning is set to play an even more transformative role, reducing the time and cost of drug development and improving patient outcomes globally. The potential is vast, and the journey has only just begun.
This article offers an excellent breakdown of the USFDA’s critical role in the drug approval process. As someone following developments in the pharmaceutical industry, I found it especially helpful in understanding how clinical trials are rigorously evaluated to ensure both safety and efficacy. It’s also a great resource for healthcare professionals looking to stay informed about regulatory standards that directly impact patient care. Looking forward to more insightful content like this!