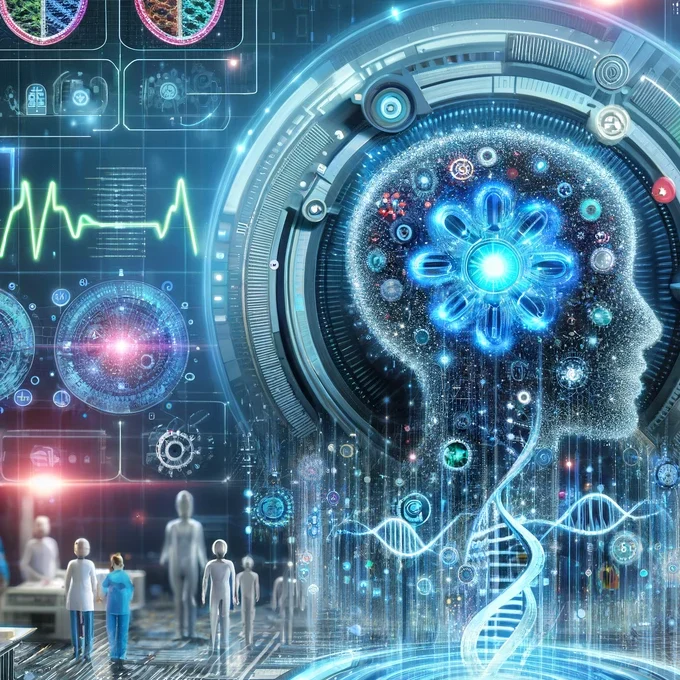
AI and Machine Learning in Healthcare Data Analytics
Welcome to the dawn of a new era in healthcare, where Artificial Intelligence (AI) and Machine Learning (ML) are not just buzzwords, but powerful tools reshaping patient care. Imagine a world where AI is the new stethoscope, making diagnoses more accurate, treatments more personalized, and healthcare data management akin to a finely-tuned orchestra. This isn’t science fiction; it’s the reality of today’s healthcare revolution.
AI and ML, akin to skilled artists, use the canvas of healthcare data to paint a future where medical errors are reduced and outcomes are improved. Like a trusted assistant, AI helps doctors by sifting through mountains of data, providing insights that the human eye might miss. ML algorithms, on the other hand, continuously learn from this data, evolving like a seasoned doctor who gains more knowledge with each patient. Together, they offer a promise of healthcare that’s not just reactive, but proactive and predictive.
This transformative journey into AI and ML in healthcare is not just a leap into the future of medicine; it’s a stride towards a world where technology and human expertise join forces to create a healthier society.
Understanding Healthcare Data: The Foundation of AI Applications
Healthcare data is the lifeblood of AI and ML applications. It’s as diverse as the human body, encompassing everything from patient records and laboratory tests to imaging and genomic data. This data is not just vast; it’s a complex tapestry of information that provides the foundation for AI-driven healthcare advancements.
Consider the electronic health record (EHR) – a digital diary of a patient’s medical history. EHRs are goldmines of data, rich with insights waiting to be unlocked by AI. Then there’s medical imaging, where AI acts like an eagle-eyed radiologist, spotting nuances in X-rays or MRIs that herald the early signs of disease. Genomic data, the blueprint of life, offers another layer of complexity. AI and ML dive into this genetic puzzle, uncovering links between genes and diseases, paving the way for personalized medicine.
These real-world examples underscore the potential of AI and ML in healthcare. They show how AI can turn this intricate, multifaceted data into actionable insights, leading to better patient outcomes and a transformed healthcare landscape.
The Mechanics of Machine Learning: How Algorithms Learn from Health Data
Imagine machine learning algorithms as curious apprentices in a healthcare setting. They keenly observe patterns in data, just as a medical student learns to recognize symptoms of diseases. These algorithms, from neural networks to decision trees, sift through vast amounts of health data, extracting valuable insights. Think of a neural network as a complex web of neurons in the human brain, each connection fine-tuning itself to better understand patient data. On the other hand, decision trees mimic a doctor’s diagnostic process, branching out into different symptoms and signs to reach a diagnosis.
Unsupervised learning, another key player, resembles a detective unearthing hidden patterns without explicit guidance. It’s like discovering unknown genetic markers in patients that could be pivotal in disease treatment. However, the success of these learning algorithms hinges on the quality of data they are fed. Accurate, diverse, and extensive datasets lead to more reliable and applicable medical insights.
Yet, with great power comes great responsibility. As these algorithms delve into sensitive health records, maintaining data privacy and security is paramount. Adhering to stringent data protection regulations and ethical standards is not just necessary but a moral obligation in this digital healthcare era.
Revolutionizing Diagnosis and Treatment: AI in Action
AI’s role in transforming healthcare is no less than revolutionary. In oncology, AI algorithms are the new pathfinders, identifying subtle patterns in imaging that escape the human eye. They detect tumors earlier and with more precision, paving the way for timely and effective treatment. Personalized treatment plans, tailored to the genetic makeup of individual patients, are no longer a distant dream, thanks to AI’s deep learning capabilities.
In cardiology, AI is a game-changer. Algorithms analyze ECG patterns, predicting heart attacks even before patients show symptoms. This predictive power of AI enables preventive healthcare, saving countless lives. Recently, AI-driven models in heart disease management have shown remarkable accuracy, rivaling seasoned cardiologists.
These case studies are just the tip of the iceberg. AI’s potential in diagnosing diseases, predicting outcomes, and personalizing treatments is boundless. It’s reshaping healthcare, making it more proactive, personalized, and predictive. As AI continues to evolve, its integration into healthcare promises a future where disease diagnosis is quicker, treatments are more effective, and patient outcomes are significantly improved.
- General Diagnostic Accuracy: A study highlighted in Medical News Today revealed that AI can correctly diagnose disease in 87% of cases, compared to an 86% accuracy rate for healthcare professionals. This indicates a very high level of proficiency for AI in medical diagnostics.
- AI in Pediatric Diagnosis: According to a study reported in Nature Medicine, AI has demonstrated its prowess in diagnosing childhood diseases, showing accuracy comparable to that of two physicians combined. This finding is significant as it underscores AI’s potential in pediatric healthcare, a field where accurate and early diagnosis can have a profound impact on treatment outcomes.
- AI in Dermatology: Stanford University conducted a study illustrating AI’s ability to detect skin cancer with accuracy equal to that of experienced dermatologists. This is a crucial development, especially considering the global rise in skin cancer cases and the need for early, accurate diagnosis.
- Ovarian Cancer Prediction: AI has achieved a notable 95% accuracy rate in predicting ovarian cancer, according to a study published by Nature. Given the typically late detection of this disease, AI’s high accuracy in early prediction could be a game-changer in treatment and survival rates.
- AI in Radiology: The American Hospital Association notes that AI’s most significant application in diagnostics has been in imaging, particularly in detecting lung nodules on CT scans and breast imaging. The Food and Drug Administration (FDA) has approved nearly 400 AI algorithms for use in radiology, reflecting the widespread acceptance and trust in AI’s diagnostic capabilities in this field.
- Improving Patient Safety: AI is also making strides in improving patient safety by enhancing error detection, patient stratification, and drug delivery management. These developments are particularly evident in radiology, where AI is used to detect early-stage cancers and other conditions that might be missed during manual examination, thereby potentially saving lives and improving long-term health outcomes.
Challenges and Ethical Considerations in AI-Driven Healthcare
Incorporating AI into healthcare ushers in a new era of innovation but also presents significant challenges and ethical dilemmas. Central to these is data privacy. Healthcare data is incredibly personal, and safeguarding it is paramount. The integration of AI necessitates robust data protection strategies to prevent breaches that could have far-reaching implications for patient confidentiality.
Another critical concern is the risk of algorithmic bias. AI systems learn from datasets, and if these datasets are biased, the AI’s decisions will reflect that bias. This can lead to unequal treatment and outcomes in patient care, exacerbating existing healthcare disparities. Ensuring diverse, representative data is essential to mitigate this risk.
Moreover, ethical considerations in AI-driven healthcare are complex. The decision-making process of AI algorithms can be opaque, leading to concerns about accountability, especially in cases of misdiagnosis or treatment errors. Establishing transparent AI systems and clear accountability guidelines is crucial for maintaining trust in healthcare AI.
A human-centric approach in AI implementation is vital. AI should augment, not replace, human decision-making in healthcare. This involves maintaining a balance where AI provides insights and efficiencies while healthcare professionals retain ultimate control over patient care decisions.
In conclusion, while AI has transformative potential in healthcare, addressing these challenges is necessary for its successful and ethical implementation. By prioritizing data privacy, actively working to eliminate algorithmic bias, adhering to ethical standards, and maintaining a human-centric approach, AI can be harnessed to significantly enhance healthcare outcomes.
I’m often to blogging and i really appreciate your content. The article has actually peaks my interest. I’m going to bookmark your web site and maintain checking for brand spanking new information.